Fortunato, Francesca
(2018)
High-dimensional and one-class classification, [Dissertation thesis], Alma Mater Studiorum Università di Bologna.
Dottorato di ricerca in
Scienze statistiche, 30 Ciclo. DOI 10.6092/unibo/amsdottorato/8412.
Documenti full-text disponibili:
![ThesisMain.pdf [thumbnail of ThesisMain.pdf]](https://amsdottorato.unibo.it/8412/1.hassmallThumbnailVersion/ThesisMain.pdf) 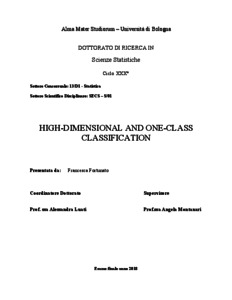 Anteprima |
Documento PDF (English)
- Richiede un lettore di PDF come Xpdf o Adobe Acrobat Reader
Disponibile con Licenza: Salvo eventuali più ampie autorizzazioni dell'autore, la tesi può essere liberamente consultata e può essere effettuato il salvataggio e la stampa di una copia per fini strettamente personali di studio, di ricerca e di insegnamento, con espresso divieto di qualunque utilizzo direttamente o indirettamente commerciale. Ogni altro diritto sul materiale è riservato.
Download (7MB)
| Anteprima
|
Abstract
When dealing with high-dimensional data and, in particular, when the number of attributes p is large comparatively to the sample size n, several classification methods cannot be applied. Fisher's linear discriminant rule or the quadratic discriminant one are unfeasible, as the inverse of the involved covariance matrices cannot be computed. A recent approach to overcome this problem is based on Random Projections (RPs), which have emerged as a powerful method for dimensionality reduction. In 2017, Cannings and Samworth introduced the RP method in the ensemble context to extend to the high-dimensional domain classification methods originally designed for low-dimensional data. Although the RP ensemble classifier allows improving classification accuracy, it may still include redundant information. Moreover, differently from other ensemble classifiers (e.g. Random Forest), it does not provide any insight on the actual classification importance of the input features. To account for these aspects, in the first part of this thesis, we investigate two new directions of the RP ensemble classifier. Firstly, combining the original idea of using the Multiplicative Binomial distribution as the reference model to describe and predict the ensemble accuracy and an important result on such distribution, we introduce a stepwise strategy for post-pruning (called Ensemble Selection Algorithm). Secondly, we propose a criterion (called Variable Importance in Projection) that uses the feature coefficients in the best discriminant projections to measure the variable importance in classification. In the second part, we faced the new challenges posed by the high-dimensional data in a recently emerging classification context: one-class classification. This is a special classification task, where only one class is fully known (the target class), while the information on the others is completely missing. In particular, we address this task by using Gini's transvariation probability as a measure of typicality, aimed at identifying the best boundary around the target class.
Abstract
When dealing with high-dimensional data and, in particular, when the number of attributes p is large comparatively to the sample size n, several classification methods cannot be applied. Fisher's linear discriminant rule or the quadratic discriminant one are unfeasible, as the inverse of the involved covariance matrices cannot be computed. A recent approach to overcome this problem is based on Random Projections (RPs), which have emerged as a powerful method for dimensionality reduction. In 2017, Cannings and Samworth introduced the RP method in the ensemble context to extend to the high-dimensional domain classification methods originally designed for low-dimensional data. Although the RP ensemble classifier allows improving classification accuracy, it may still include redundant information. Moreover, differently from other ensemble classifiers (e.g. Random Forest), it does not provide any insight on the actual classification importance of the input features. To account for these aspects, in the first part of this thesis, we investigate two new directions of the RP ensemble classifier. Firstly, combining the original idea of using the Multiplicative Binomial distribution as the reference model to describe and predict the ensemble accuracy and an important result on such distribution, we introduce a stepwise strategy for post-pruning (called Ensemble Selection Algorithm). Secondly, we propose a criterion (called Variable Importance in Projection) that uses the feature coefficients in the best discriminant projections to measure the variable importance in classification. In the second part, we faced the new challenges posed by the high-dimensional data in a recently emerging classification context: one-class classification. This is a special classification task, where only one class is fully known (the target class), while the information on the others is completely missing. In particular, we address this task by using Gini's transvariation probability as a measure of typicality, aimed at identifying the best boundary around the target class.
Tipologia del documento
Tesi di dottorato
Autore
Fortunato, Francesca
Supervisore
Dottorato di ricerca
Ciclo
30
Coordinatore
Settore disciplinare
Settore concorsuale
Parole chiave
Random-projection; One-class classification; Discriminant Analysis
URN:NBN
DOI
10.6092/unibo/amsdottorato/8412
Data di discussione
8 Maggio 2018
URI
Altri metadati
Tipologia del documento
Tesi di dottorato
Autore
Fortunato, Francesca
Supervisore
Dottorato di ricerca
Ciclo
30
Coordinatore
Settore disciplinare
Settore concorsuale
Parole chiave
Random-projection; One-class classification; Discriminant Analysis
URN:NBN
DOI
10.6092/unibo/amsdottorato/8412
Data di discussione
8 Maggio 2018
URI
Statistica sui download
Gestione del documento: