Borghesi, Andrea
(2017)
Power-Aware Job Dispatching in High Performance Computing Systems, [Dissertation thesis], Alma Mater Studiorum Università di Bologna.
Dottorato di ricerca in
Computer science and engineering, 29 Ciclo. DOI 10.6092/unibo/amsdottorato/7982.
Documenti full-text disponibili:
![[img]](http://amsdottorato.unibo.it/7982/1.hassmallThumbnailVersion/master.pdf) 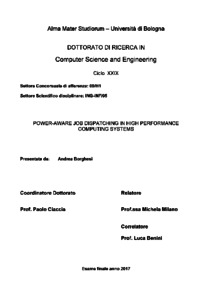 Anteprima |
|
Documento PDF (English)
- Richiede un lettore di PDF come Xpdf o Adobe Acrobat Reader
Disponibile con Licenza: Salvo eventuali più ampie autorizzazioni dell'autore, la tesi può essere liberamente consultata e può essere effettuato il salvataggio e la stampa di una copia per fini strettamente personali di studio, di ricerca e di insegnamento, con espresso divieto di qualunque utilizzo direttamente o indirettamente commerciale. Ogni altro diritto sul materiale è riservato.
Download (4MB)
| Anteprima
|
Abstract
This works deals with the power-aware job dispatching problem in supercomputers; broadly speaking the dispatching consists of assigning finite capacity resources to a set of activities, with a special concern toward power and energy efficient solutions. We introduce novel optimization approaches to address its multiple aspects.
The proposed techniques have a broad application range but are aimed at applications in the field of High Performance Computing (HPC) systems.
Devising a power-aware HPC job dispatcher is a complex, where contrasting goals must be satisfied. Furthermore, the online nature of the problem request that solutions must be computed in real time respecting stringent limits. This aspect historically discouraged the usage of exact methods and favouring instead the adoption of heuristic techniques. The application of optimization approaches to the dispatching task is still an unexplored area of research and can drastically improve the performance of HPC systems.
In this work we tackle the job dispatching problem on a real HPC machine, the Eurora supercomputer hosted at the Cineca research center, Bologna. We propose a Constraint Programming (CP) model that outperforms the dispatching software currently in use. An essential element to take power-aware decisions during the job dispatching phase is the possibility to estimate jobs power consumptions before their execution. To this end, we applied Machine Learning techniques to create a prediction model that was trained and tested on the Euora supercomputer, showing a great prediction accuracy. Then we finally develop a power-aware solution, considering the same target machine, and we devise different approaches to solve the dispatching problem while curtailing the power consumption of the whole system under a given threshold. We proposed a heuristic technique and a CP/heuristic hybrid method, both able to solve practical size instances and outperform the current state-of-the-art techniques.
Abstract
This works deals with the power-aware job dispatching problem in supercomputers; broadly speaking the dispatching consists of assigning finite capacity resources to a set of activities, with a special concern toward power and energy efficient solutions. We introduce novel optimization approaches to address its multiple aspects.
The proposed techniques have a broad application range but are aimed at applications in the field of High Performance Computing (HPC) systems.
Devising a power-aware HPC job dispatcher is a complex, where contrasting goals must be satisfied. Furthermore, the online nature of the problem request that solutions must be computed in real time respecting stringent limits. This aspect historically discouraged the usage of exact methods and favouring instead the adoption of heuristic techniques. The application of optimization approaches to the dispatching task is still an unexplored area of research and can drastically improve the performance of HPC systems.
In this work we tackle the job dispatching problem on a real HPC machine, the Eurora supercomputer hosted at the Cineca research center, Bologna. We propose a Constraint Programming (CP) model that outperforms the dispatching software currently in use. An essential element to take power-aware decisions during the job dispatching phase is the possibility to estimate jobs power consumptions before their execution. To this end, we applied Machine Learning techniques to create a prediction model that was trained and tested on the Euora supercomputer, showing a great prediction accuracy. Then we finally develop a power-aware solution, considering the same target machine, and we devise different approaches to solve the dispatching problem while curtailing the power consumption of the whole system under a given threshold. We proposed a heuristic technique and a CP/heuristic hybrid method, both able to solve practical size instances and outperform the current state-of-the-art techniques.
Tipologia del documento
Tesi di dottorato
Autore
Borghesi, Andrea
Supervisore
Co-supervisore
Dottorato di ricerca
Ciclo
29
Coordinatore
Settore disciplinare
Settore concorsuale
Parole chiave
High Performance Computing
Constraint Programming
Machine Learning
Scheduling & Allocation
Power Prediction
URN:NBN
DOI
10.6092/unibo/amsdottorato/7982
Data di discussione
15 Maggio 2017
URI
Altri metadati
Tipologia del documento
Tesi di dottorato
Autore
Borghesi, Andrea
Supervisore
Co-supervisore
Dottorato di ricerca
Ciclo
29
Coordinatore
Settore disciplinare
Settore concorsuale
Parole chiave
High Performance Computing
Constraint Programming
Machine Learning
Scheduling & Allocation
Power Prediction
URN:NBN
DOI
10.6092/unibo/amsdottorato/7982
Data di discussione
15 Maggio 2017
URI
Statistica sui download
Gestione del documento: