Banchelli, Federico
(2017)
A Recursive Partitioning Approach to Hospital Case Mix Classification, [Dissertation thesis], Alma Mater Studiorum Università di Bologna.
Dottorato di ricerca in
Scienze statistiche, 29 Ciclo. DOI 10.6092/unibo/amsdottorato/7776.
Documenti full-text disponibili:
![Banchelli_Federico_Tesi.pdf [thumbnail of Banchelli_Federico_Tesi.pdf]](https://amsdottorato.unibo.it/7776/1.hassmallThumbnailVersion/Banchelli_Federico_Tesi.pdf) 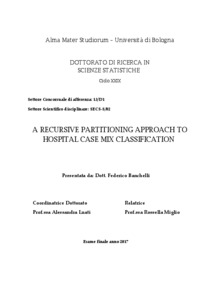 Anteprima |
Documento PDF (English)
- Richiede un lettore di PDF come Xpdf o Adobe Acrobat Reader
Disponibile con Licenza: Salvo eventuali più ampie autorizzazioni dell'autore, la tesi può essere liberamente consultata e può essere effettuato il salvataggio e la stampa di una copia per fini strettamente personali di studio, di ricerca e di insegnamento, con espresso divieto di qualunque utilizzo direttamente o indirettamente commerciale. Ogni altro diritto sul materiale è riservato.
Download (5MB)
| Anteprima
|
Abstract
The present dissertation was focused on the study and development of a clinical data mining methodology for hospital case mix iso-resource classification. Several recursive partitioning methodologies were applied on Emilia-Romagna Region hospital discharge database. Here, the need for developing several alternative iso-resource subgroups was a critical point in the development of case mix classification systems, due to the presence of clinical coherence requirements.
Two major classes of trees were assessed: constant-fit trees and model-based trees, with a particular focus on the latter class, which peculiarity is to fit regression models in the nodes of the tree. After an extensive literature review, the traditional regression tree (constant-fit) and four model-based tree algorithms were assessed: two modifications of the Model-Based Recursive Partitioning (MOB) algorithm which were given additional flexibility by performing a within-node model selection step, respectively using count regression and continuous response regression GLMs; a two-step composite algorithm which fits regression trees and models in terminal nodes; quantile-model-based regression trees, by means of the Generalized Unbiased Interaction Detection and Estimation (GUIDE) algorithm.
These algorithms were compared under several points of view. Statistical performance, measured via bootstrap out-of-bag performance curves, was in favor of model-based trees, while, among them, competing performances were found. Implications for the design of hospital case mix classification systems were also evaluated, since the two classes of trees can be conceptually linked to different refunding schemes. Moreover, application and advantages of two different ensemble methods were discussed.
All the recursive partitioning methods employed resulted in the definition of iso-resource clinically similar subgroups of patients. Different interpretations were given to these alternative subgroups, due to differences in the rationale of the various splitting criteria. In particular, model-based trees identified subgroups with differential effects of patient’s age and clinical severity on resource consumption, here measured with hospital length of stay.
Abstract
The present dissertation was focused on the study and development of a clinical data mining methodology for hospital case mix iso-resource classification. Several recursive partitioning methodologies were applied on Emilia-Romagna Region hospital discharge database. Here, the need for developing several alternative iso-resource subgroups was a critical point in the development of case mix classification systems, due to the presence of clinical coherence requirements.
Two major classes of trees were assessed: constant-fit trees and model-based trees, with a particular focus on the latter class, which peculiarity is to fit regression models in the nodes of the tree. After an extensive literature review, the traditional regression tree (constant-fit) and four model-based tree algorithms were assessed: two modifications of the Model-Based Recursive Partitioning (MOB) algorithm which were given additional flexibility by performing a within-node model selection step, respectively using count regression and continuous response regression GLMs; a two-step composite algorithm which fits regression trees and models in terminal nodes; quantile-model-based regression trees, by means of the Generalized Unbiased Interaction Detection and Estimation (GUIDE) algorithm.
These algorithms were compared under several points of view. Statistical performance, measured via bootstrap out-of-bag performance curves, was in favor of model-based trees, while, among them, competing performances were found. Implications for the design of hospital case mix classification systems were also evaluated, since the two classes of trees can be conceptually linked to different refunding schemes. Moreover, application and advantages of two different ensemble methods were discussed.
All the recursive partitioning methods employed resulted in the definition of iso-resource clinically similar subgroups of patients. Different interpretations were given to these alternative subgroups, due to differences in the rationale of the various splitting criteria. In particular, model-based trees identified subgroups with differential effects of patient’s age and clinical severity on resource consumption, here measured with hospital length of stay.
Tipologia del documento
Tesi di dottorato
Autore
Banchelli, Federico
Supervisore
Dottorato di ricerca
Ciclo
29
Coordinatore
Settore disciplinare
Settore concorsuale
Parole chiave
Model-based trees ; Recursive partitioning ; Clinical data mining ; Diagnosis-related groups ; Length of stay ; Patient Classification Systems
URN:NBN
DOI
10.6092/unibo/amsdottorato/7776
Data di discussione
17 Febbraio 2017
URI
Altri metadati
Tipologia del documento
Tesi di dottorato
Autore
Banchelli, Federico
Supervisore
Dottorato di ricerca
Ciclo
29
Coordinatore
Settore disciplinare
Settore concorsuale
Parole chiave
Model-based trees ; Recursive partitioning ; Clinical data mining ; Diagnosis-related groups ; Length of stay ; Patient Classification Systems
URN:NBN
DOI
10.6092/unibo/amsdottorato/7776
Data di discussione
17 Febbraio 2017
URI
Statistica sui download
Gestione del documento: