Poggi, Matteo
(2018)
Machine Learning techniques applied to stereo vision, [Dissertation thesis], Alma Mater Studiorum Università di Bologna.
Dottorato di ricerca in
Computer science and engineering, 30 Ciclo. DOI 10.6092/unibo/amsdottorato/8444.
Documenti full-text disponibili:
![thesis.pdf [thumbnail of thesis.pdf]](https://amsdottorato.unibo.it/8444/1.hassmallThumbnailVersion/thesis.pdf) 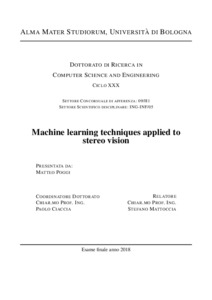 Anteprima |
Documento PDF (English)
- Richiede un lettore di PDF come Xpdf o Adobe Acrobat Reader
Disponibile con Licenza: Salvo eventuali più ampie autorizzazioni dell'autore, la tesi può essere liberamente consultata e può essere effettuato il salvataggio e la stampa di una copia per fini strettamente personali di studio, di ricerca e di insegnamento, con espresso divieto di qualunque utilizzo direttamente o indirettamente commerciale. Ogni altro diritto sul materiale è riservato.
Download (51MB)
| Anteprima
|
Abstract
Stereo is a popular technique enabling fast and dense depth estimation from two or more images.
Its success is mainly due to its easiness of deployment, requiring only a couple or multiple synchronized image sensors, accurately calibrated to solve the matching problem between pixels on one of the images (named reference) and the other (named target). The absence of active technologies (e.g. pattern projection, laser scanners etc..) make this solution deployable on almost every scenario. Despite the wide literature concerning stereo, it still represents an open problem because of very challenging conditions such as poor illumination, reflective surfaces, occlusions and other elements occurring in real environments.
Two main trends in stereo vision acquired popularity in the last years: confidence estimation and machine learning. Both proved to be very effective, pushing forward the state-of-the-art of dense disparity estimation.
In this thesis, we combine these two trends to improve both confidence estimation and disparity inference, by defining more effective and easier to deploy confidence measures and proposing new approaches to leverage on them for more accurate depth prediction.
All the experiments are validated on three popular datasets, KITTI 2012, KITTI 2015 and Middlebury v3, following the commonly adopted methodologies and protocol to compare our proposals with previous works representing the state-of-the-art in stereo vision.
Abstract
Stereo is a popular technique enabling fast and dense depth estimation from two or more images.
Its success is mainly due to its easiness of deployment, requiring only a couple or multiple synchronized image sensors, accurately calibrated to solve the matching problem between pixels on one of the images (named reference) and the other (named target). The absence of active technologies (e.g. pattern projection, laser scanners etc..) make this solution deployable on almost every scenario. Despite the wide literature concerning stereo, it still represents an open problem because of very challenging conditions such as poor illumination, reflective surfaces, occlusions and other elements occurring in real environments.
Two main trends in stereo vision acquired popularity in the last years: confidence estimation and machine learning. Both proved to be very effective, pushing forward the state-of-the-art of dense disparity estimation.
In this thesis, we combine these two trends to improve both confidence estimation and disparity inference, by defining more effective and easier to deploy confidence measures and proposing new approaches to leverage on them for more accurate depth prediction.
All the experiments are validated on three popular datasets, KITTI 2012, KITTI 2015 and Middlebury v3, following the commonly adopted methodologies and protocol to compare our proposals with previous works representing the state-of-the-art in stereo vision.
Tipologia del documento
Tesi di dottorato
Autore
Poggi, Matteo
Supervisore
Dottorato di ricerca
Ciclo
30
Coordinatore
Settore disciplinare
Settore concorsuale
Parole chiave
Computer vision, stereo, machine learning, deep learning
URN:NBN
DOI
10.6092/unibo/amsdottorato/8444
Data di discussione
20 Aprile 2018
URI
Altri metadati
Tipologia del documento
Tesi di dottorato
Autore
Poggi, Matteo
Supervisore
Dottorato di ricerca
Ciclo
30
Coordinatore
Settore disciplinare
Settore concorsuale
Parole chiave
Computer vision, stereo, machine learning, deep learning
URN:NBN
DOI
10.6092/unibo/amsdottorato/8444
Data di discussione
20 Aprile 2018
URI
Statistica sui download
Gestione del documento: