Gallinucci, Enrico
(2017)
Business Intelligence on Non-Conventional Data, [Dissertation thesis], Alma Mater Studiorum Università di Bologna.
Dottorato di ricerca in
Computer science and engineering, 29 Ciclo. DOI 10.6092/unibo/amsdottorato/7863.
Documenti full-text disponibili:
![[img]](http://amsdottorato.unibo.it/7863/1.hassmallThumbnailVersion/Phd%20thesis%20Gallinucci%20Enrico.pdf) 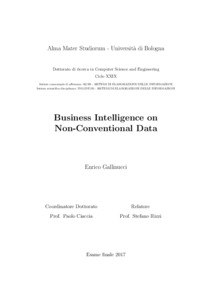 Anteprima |
|
Documento PDF (English)
- Richiede un lettore di PDF come Xpdf o Adobe Acrobat Reader
Disponibile con Licenza: Salvo eventuali più ampie autorizzazioni dell'autore, la tesi può essere liberamente consultata e può essere effettuato il salvataggio e la stampa di una copia per fini strettamente personali di studio, di ricerca e di insegnamento, con espresso divieto di qualunque utilizzo direttamente o indirettamente commerciale. Ogni altro diritto sul materiale è riservato.
Download (18MB)
| Anteprima
|
Abstract
The revolution in digital communications witnessed over the last decade had a significant impact on the world of Business Intelligence (BI). In the big data era, the amount and diversity of data that can be collected and analyzed for the decision-making process transcends the restricted and structured set of internal data that BI systems are conventionally limited to. This thesis investigates the unique challenges imposed by three specific categories of non-conventional data: social data, linked data and schemaless data. Social data comprises the user-generated contents published through websites and social media, which can provide a fresh and timely perception about people’s tastes and opinions. In Social BI (SBI), the analysis focuses on topics, meant as specific concepts of interest within the subject area. In this context, this thesis proposes meta-star, an alternative strategy to the traditional star-schema for modeling hierarchies of topics to enable OLAP analyses. The thesis also presents an architectural framework of a real SBI project and a cross-disciplinary benchmark for SBI. Linked data employ the Resource Description Framework (RDF) to provide a public network of interlinked, structured, cross-domain knowledge. In this context, this thesis proposes an interactive and collaborative approach to build aggregation hierarchies from linked data. Schemaless data refers to the storage of data in NoSQL databases that do not force a predefined schema, but let database instances embed their own local schemata. In this context, this thesis proposes an approach to determine the schema profile of a document-based database; the goal is to facilitate users in a schema-on-read analysis process by understanding the rules that drove the usage of the different schemata. A final and complementary contribution of this thesis is an innovative technique in the field of recommendation systems to overcome user disorientation in the analysis of a large and heterogeneous wealth of data.
Abstract
The revolution in digital communications witnessed over the last decade had a significant impact on the world of Business Intelligence (BI). In the big data era, the amount and diversity of data that can be collected and analyzed for the decision-making process transcends the restricted and structured set of internal data that BI systems are conventionally limited to. This thesis investigates the unique challenges imposed by three specific categories of non-conventional data: social data, linked data and schemaless data. Social data comprises the user-generated contents published through websites and social media, which can provide a fresh and timely perception about people’s tastes and opinions. In Social BI (SBI), the analysis focuses on topics, meant as specific concepts of interest within the subject area. In this context, this thesis proposes meta-star, an alternative strategy to the traditional star-schema for modeling hierarchies of topics to enable OLAP analyses. The thesis also presents an architectural framework of a real SBI project and a cross-disciplinary benchmark for SBI. Linked data employ the Resource Description Framework (RDF) to provide a public network of interlinked, structured, cross-domain knowledge. In this context, this thesis proposes an interactive and collaborative approach to build aggregation hierarchies from linked data. Schemaless data refers to the storage of data in NoSQL databases that do not force a predefined schema, but let database instances embed their own local schemata. In this context, this thesis proposes an approach to determine the schema profile of a document-based database; the goal is to facilitate users in a schema-on-read analysis process by understanding the rules that drove the usage of the different schemata. A final and complementary contribution of this thesis is an innovative technique in the field of recommendation systems to overcome user disorientation in the analysis of a large and heterogeneous wealth of data.
Tipologia del documento
Tesi di dottorato
Autore
Gallinucci, Enrico
Supervisore
Dottorato di ricerca
Ciclo
29
Coordinatore
Settore disciplinare
Settore concorsuale
Parole chiave
Business intelligence
Multidimensional modeling
Data warehouse design
Social media
User-generated content
Social BI
Sentiment analysis
OLAP
Exploratory BI
Linked data
NoSQL
Document-oriented databases
Schema discovery
Query recommendations
Benchmarking
URN:NBN
DOI
10.6092/unibo/amsdottorato/7863
Data di discussione
15 Maggio 2017
URI
Altri metadati
Tipologia del documento
Tesi di dottorato
Autore
Gallinucci, Enrico
Supervisore
Dottorato di ricerca
Ciclo
29
Coordinatore
Settore disciplinare
Settore concorsuale
Parole chiave
Business intelligence
Multidimensional modeling
Data warehouse design
Social media
User-generated content
Social BI
Sentiment analysis
OLAP
Exploratory BI
Linked data
NoSQL
Document-oriented databases
Schema discovery
Query recommendations
Benchmarking
URN:NBN
DOI
10.6092/unibo/amsdottorato/7863
Data di discussione
15 Maggio 2017
URI
Statistica sui download
Gestione del documento: