Ayetiran, Eniafe Festus
(2017)
A Combined Unsupervised Technique for Automatic Classification in Electronic Discovery, [Dissertation thesis], Alma Mater Studiorum Università di Bologna.
Dottorato di ricerca in
Law, science and technology, 28 Ciclo. DOI 10.6092/unibo/amsdottorato/7789.
Documenti full-text disponibili:
![[img]](http://amsdottorato.unibo.it/7789/1.hassmallThumbnailVersion/AYETIRAN_ENIAFE_FESTUS_TESI.pdf) 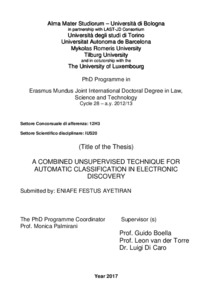 Anteprima |
|
Documento PDF (English)
- Richiede un lettore di PDF come Xpdf o Adobe Acrobat Reader
Disponibile con Licenza: Salvo eventuali più ampie autorizzazioni dell'autore, la tesi può essere liberamente consultata e può essere effettuato il salvataggio e la stampa di una copia per fini strettamente personali di studio, di ricerca e di insegnamento, con espresso divieto di qualunque utilizzo direttamente o indirettamente commerciale. Ogni altro diritto sul materiale è riservato.
Download (1MB)
| Anteprima
|
Abstract
In this work, we present an automated unsupervised approach for retrieval/classification in eDiscovery. This approach is an ad hoc retrieval which creates a representative for each original document in the collection using latent dirichlet allocation (LDA) model with Gibbs sampling and explores word sense disambiguation (WSD) to give these representative documents and queries deeper meanings for distributional semantic similarity. The word sense disambiguation technique by itself is a hybrid algorithm derived from the modified version of the original Lesk algorithm and the Jiang & Conrath similarity measure.
Evaluation was carried out on this technique using the TREC legal track. Results and observations are discussed in chapter 8. We conclude that WSD can improve ad hoc retrieval effectiveness. Finally, we suggest further on efficient algorithms for word sense disambiguation which can further improve retrieval effectiveness if applied to original document collections against using representative collections.
Abstract
In this work, we present an automated unsupervised approach for retrieval/classification in eDiscovery. This approach is an ad hoc retrieval which creates a representative for each original document in the collection using latent dirichlet allocation (LDA) model with Gibbs sampling and explores word sense disambiguation (WSD) to give these representative documents and queries deeper meanings for distributional semantic similarity. The word sense disambiguation technique by itself is a hybrid algorithm derived from the modified version of the original Lesk algorithm and the Jiang & Conrath similarity measure.
Evaluation was carried out on this technique using the TREC legal track. Results and observations are discussed in chapter 8. We conclude that WSD can improve ad hoc retrieval effectiveness. Finally, we suggest further on efficient algorithms for word sense disambiguation which can further improve retrieval effectiveness if applied to original document collections against using representative collections.
Tipologia del documento
Tesi di dottorato
Autore
Ayetiran, Eniafe Festus
Supervisore
Co-supervisore
Dottorato di ricerca
Scuola di dottorato
Scienze giuridiche
Ciclo
28
Coordinatore
Settore disciplinare
Settore concorsuale
Parole chiave
word sense disambiguation, topic modelling, Lesk, latent dirichlet allocation model, unsupervised, eDiscovery
URN:NBN
DOI
10.6092/unibo/amsdottorato/7789
Data di discussione
31 Gennaio 2017
URI
Altri metadati
Tipologia del documento
Tesi di dottorato
Autore
Ayetiran, Eniafe Festus
Supervisore
Co-supervisore
Dottorato di ricerca
Scuola di dottorato
Scienze giuridiche
Ciclo
28
Coordinatore
Settore disciplinare
Settore concorsuale
Parole chiave
word sense disambiguation, topic modelling, Lesk, latent dirichlet allocation model, unsupervised, eDiscovery
URN:NBN
DOI
10.6092/unibo/amsdottorato/7789
Data di discussione
31 Gennaio 2017
URI
Statistica sui download
Gestione del documento: